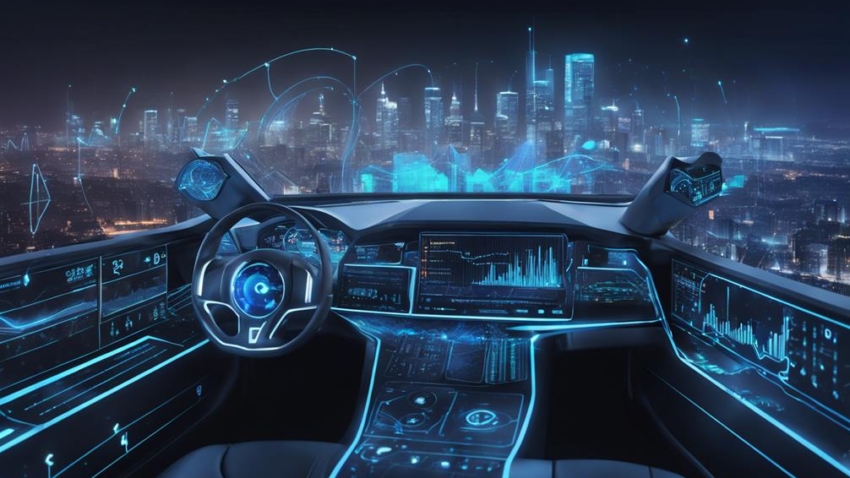
Strategies for Dominating Your Market With Advanced Analytics
To dominate their market, organizations must harness the power of advanced analytics to inform their strategies, reveal hidden insights, and drive data-driven decision-making. This requires embracing a data-driven culture, identifying key performance indicators, and leveraging AI to discover patterns and relationships in data. Advanced segmentation strategies, predictive modeling, and data visualization best practices can also help reveal growth opportunities. By balancing precision with actionability and measuring ROI on analytics investments, organizations can accelerate decision-making agility and drive significant business outcomes. Now, discover the strategies and innovative approaches that can propel your organization to market dominance.
Building a Data-Driven Culture
Embracing a data-driven culture is a pivotal step in the analytics journey, as it fosters an environment where data becomes the cornerstone of decision-making, driving business outcomes and strategic growth. This cultural shift is essential in today's data-rich landscape, where organizations that fail to adapt risk being left behind. A data-driven culture is built on the foundation of data literacy, where employees possess the skills to collect, analyze, and interpret data to inform business decisions.
Achieving data literacy requires a concerted effort to upskill and reskill the workforce, ensuring that data analysis is democratized across the organization. This, in turn, enables a cultural shift, where data becomes the language of business, and insights are used to drive strategic growth. By cultivating a data-driven culture, organizations can break down silos, foster collaboration, and create a unified understanding of business objectives.
A data-driven culture also promotes a culture of experimentation, where data is used to test hypotheses, and insights are used to refine business strategies. This iterative approach enables organizations to adapt quickly to changing market conditions, stay ahead of the competition, and dominate their market. By embracing a data-driven culture, organizations can realize the full potential of advanced analytics, drive business outcomes, and achieve sustainable growth.
Identifying Key Performance Indicators
To identify key performance indicators (KPIs), organizations must first define clear business objectives that align with their overall strategy. Next, they must measure performance metrics that accurately reflect progress toward these objectives, providing a quantifiable assessment of success. By doing so, businesses can establish a foundation for data-driven decision making and optimize their operations to achieve desired outcomes.
Defining Business Objectives
Clear business objectives serve as the foundation upon which all subsequent analytics efforts are built, providing a roadmap for data-driven decision-making and strategic planning. To establish a strong foundation, it is important to define business objectives that are specific, measurable, achievable, relevant, and time-bound (SMART). Effective goal setting enables organizations to prioritize resources, allocate budget, and align efforts towards achieving key objectives.
Objective alignment is vital to make sure that all stakeholders are working towards a common goal. By establishing a clear hierarchy of objectives, organizations can cascade goals from the top down, ensuring that every department, team, and individual is working towards a shared vision. This alignment enables organizations to focus on high-impact initiatives, eliminate redundant efforts, and optimize resource allocation. By defining business objectives and aligning them with key performance indicators, organizations can create a data-driven culture that drives growth, innovation, and market dominance.
Measuring Performance Metrics
With business objectives defined and aligned, the next step is to identify the key performance indicators (KPIs) that will measure progress towards these objectives, providing a quantifiable benchmark for success. This involves selecting metrics that are relevant, measurable, and actionable, allowing for data-driven decision-making. Metric alignment is vital, as it guarantees that KPIs are directly tied to business objectives, providing a clear line of sight to goal achievement.
Data hygiene is also essential in this process, as it guarantees that data is accurate, complete, and reliable. This includes data quality checks, data normalization, and data standardization. By establishing a robust data foundation, organizations can trust their metrics and make informed decisions. Effective KPI selection and data hygiene practices enable organizations to measure performance, identify areas for improvement, and optimize resources for maximum impact. By focusing on the right metrics, organizations can drive business outcomes, achieve strategic objectives, and dominate their market.
Unlocking Hidden Insights With AI
By harnessing the power of artificial intelligence, businesses can drill down into their data to uncover patterns and relationships that would have otherwise remained hidden. This enables organizations to gain a deeper understanding of their customers, optimize operations, and identify new opportunities for growth. However, as AI becomes increasingly integral to business decision-making, it's important to prioritize AI ethics and make sure that human oversight is maintained.
Without proper safeguards, AI systems can perpetuate biases and perpetuate existing inequalities. To avoid these pitfalls, businesses must establish clear guidelines for AI development and deployment, giving priority to transparency, accountability, and fairness. Human oversight is vital in this process, as it ensures that AI-driven insights are accurate, relevant, and aligned with business objectives.
Advanced Segmentation Strategies
Sophisticated customer segmentation is a linchpin of data-driven marketing, as it empowers businesses to craft tailored experiences that resonate deeply with their target audience. By leveraging advanced analytics, organizations can move beyond traditional segmentation approaches and reveal granular insights that drive meaningful connections with customers.
One such approach is micro segmentation, which involves dividing the market into smaller, distinct groups based on nuanced characteristics, behaviors, and preferences. This level of precision enables businesses to craft highly targeted marketing campaigns that speak directly to the needs and desires of each micro segment. For instance, a luxury fashion brand might identify a micro segment of high-income, fashion-conscious individuals who prioritize sustainability, and develop marketing strategies that appeal specifically to this group.
Another powerful tool in the advanced segmentation arsenal is persona mapping. By creating detailed, data-driven profiles of ideal customers, businesses can develop a profound understanding of their motivations, pain points, and goals. This allows for the creation of highly personalized experiences that resonate deeply with target audiences. For example, a financial services company might develop a persona for a high-net-worth individual, incorporating data points such as investment goals, risk tolerance, and communication preferences. By leveraging this level of insight, businesses can develop targeted marketing strategies that drive engagement, loyalty, and ultimately, revenue growth.
Predictive Modeling for Growth
Predictive modeling is a vital component of advanced analytics, enabling businesses to forecast outcomes and make informed decisions. To guarantee the effectiveness of predictive models, it is essential to assess their performance using metrics such as accuracy, precision, and recall. By leveraging model performance metrics and adopting a data-driven decision-making approach, organizations can reveal new opportunities for growth and stay ahead of the competition.
Model Performance Metrics
Every predictive model is only as valuable as its ability to drive accurate predictions, making the selection and tracking of relevant model performance metrics essential to informing data-driven business decisions. The choice of metrics can have a profound impact on the effectiveness of a model, and therefore, it is vital to select metrics that align with the business objectives.
To guarantee peak model performance, consider the following key metrics:
- Mean Absolute Error (MAE): measures the average difference between predicted and actual values
- Mean Squared Error (MSE): calculates the average of the squared differences between predicted and actual values
- R-Squared (R²): evaluates the model's ability to explain variance in the dependent variable
- F1 Score: balances precision and recall for classification models
- Area Under the ROC Curve (AUC-ROC): assesses the model's ability to distinguish between positive and negative classes
Data-Driven Decision Making
By harnessing advanced analytics and machine learning algorithms, businesses can tap into the power of data-driven decision making, driving growth and profitability through informed strategic planning. This approach enables organizations to move beyond intuition-based decision making, instead relying on data-driven insights to guide their strategy.
Data-driven decision making is critical in today's fast-paced business environment, where speed and agility are essential for staying ahead of the competition. To achieve this, businesses must cultivate a culture of data literacy, where employees are empowered to make data-informed decisions. This requires a combination of technical skills, business acumen, and critical thinking.
Decision Type | Decision Velocity |
---|---|
Strategic | High |
Tactical | Medium |
Operational | Low |
Real-Time Analytics for Fast Action
Most organizations today recognize the importance of swift decision-making, and real-time analytics is essential for enabling this capability. The ability to respond quickly to changing market conditions, customer needs, and operational challenges is critical in today's fast-paced business environment. Real-time analytics provides the necessary insights to facilitate rapid decision-making, enabling organizations to stay ahead of the competition.
To achieve real-time analytics, organizations can leverage the following strategies:
- Flash Insights: Implementing real-time data processing and analytics platforms to generate instant insights from large datasets.
- Rapid Response: Establishing automated workflows and alerts to trigger swift action in response to changing market conditions or operational anomalies.
- Leveraging IoT sensors and edge computing to capture and analyze data in real-time, enabling instant decision-making.
- Implementing machine learning models that can analyze large datasets in real-time, providing instant predictions and recommendations.
- Utilizing cloud-based analytics platforms that can scale to meet the demands of real-time analytics, ensuring rapid processing and analysis of large datasets.
Data Visualization Best Practices
Effective data visualization requires a strategic approach to present complex insights in a clear, concise, and pivotal manner, empowering stakeholders to make informed decisions. As a vital component of advanced analytics, data visualization enables businesses to extract valuable insights from their data and drive strategic decision-making. To dominate the market, it is essential to adopt best practices in data visualization that facilitate data storytelling and communicate insights effectively.
A well-designed visual hierarchy is critical to convey complex data insights in a clear and concise manner. This involves organizing data into a logical structure, using visual elements such as colors, shapes, and sizes to guide the viewer's attention. By doing so, stakeholders can quickly identify key trends, patterns, and correlations, and make data-driven decisions with confidence.
Data storytelling is another essential aspect of effective data visualization. It involves crafting a narrative around the data insights, using visualizations to convey the story and evoke an emotional response. By presenting data in a compelling and engaging manner, businesses can inspire action, drive change, and achieve their strategic objectives. By mastering data visualization best practices, organizations can harness the full potential of their data, gain a competitive edge, and dominate their market.
Creating a Centralized Analytics Hub
A centralized analytics hub serves as the linchpin of advanced analytics, providing a unified platform for data visualization, data storytelling, and strategic decision-making. This hub is the nerve center of your analytics ecosystem, enabling data-driven insights and actionable intelligence. By creating a centralized analytics hub, organizations can break down silos, eliminate data duplication, and guarantee data consistency across the enterprise.
Here are the key benefits of a centralized analytics hub:
- Unified Data Management: A centralized analytics hub integrates data from disparate sources, creating a single source of truth and eliminating data duplication.
- Streamlined Analytics Architecture: By integrating analytics tools and platforms, organizations can reduce complexity, improve scalability, and enhance collaboration.
- Enhanced Data Governance: A centralized hub enables robust data governance, guaranteeing data quality, security, and compliance across the enterprise.
- Faster Time-to-Insight: With a unified analytics platform, organizations can accelerate data analysis, reduce latency, and improve decision-making agility.
- Improved Collaboration: A centralized analytics hub fosters collaboration across functions, enabling cross-functional teams to work together seamlessly and drive business outcomes.
Balancing Precision With Actionability
When harnessing advanced analytics to drive business decisions, it's vital to strike a balance between precision and actionability. High-quality data is essential to generating reliable insights, but it's equally important to prioritize practicality and guarantee that insights are actionable and inform meaningful business decisions. By finding this balance, organizations can unleash the full potential of their analytics capabilities and drive significant business outcomes.
Data Quality Matters
Every business decision relies on data, but only high-quality data can provide the precision and reliability necessary to drive actionable insights. Data quality is a critical component of advanced analytics, as it directly impacts the accuracy and relevance of insights generated. To guarantee data quality, organizations must prioritize data profiling, which involves analyzing data to identify patterns, trends, and anomalies. This process helps to pinpoint errors, inconsistencies, and inaccuracies that can compromise data quality.
Additionally, implementing data governance policies and procedures is essential to uphold data quality over time. This includes establishing clear data management roles, responsibilities, and processes to ensure data is accurately captured, stored, and utilized.
Some key considerations for upholding data quality include:
- Conducting regular data audits to identify and correct errors
- Implementing data validation rules to prevent incorrect data entry
- Establishing data quality metrics to track and measure data quality
- Providing training and resources to make sure data is accurately captured and utilized
- Continuously monitoring and refining data governance policies and procedures
Actionable Insights Only
In today's data-driven landscape, organizations must strike a delicate balance between precision and actionability, as insights that are overly precise but lack relevance to business objectives are of little value. Actionable insights are the lifeblood of data-driven decision-making, and companies that fail to prioritize them risk drowning in a sea of irrelevant data. To avoid this, businesses must focus on generating insights that are both precise and actionable.
Effective data storytelling is key to achieving this balance. By presenting complex data insights in a clear, concise, and compelling narrative, organizations can make certain that stakeholders understand the relevance and impact of the data on business objectives. Insight prioritization is also vital, as it enables companies to focus on the most critical insights that drive business value. By prioritizing actionable insights, organizations can expedite decision-making, optimize resources, and drive business growth. By striking the right balance between precision and actionability, companies can unleash the full potential of their data and gain a competitive edge in the market.
Precision Vs. Practicality
While actionable insights are the backbone of data-driven decision-making, the pursuit of precision must be tempered by practical considerations, lest organizations become mired in analysis paralysis. In the quest for mathematical optimality, it's essential to acknowledge real-world tradeoffs. The pursuit of precision can lead to diminishing returns, where incremental gains in accuracy come at the cost of increased complexity, computational power, and time.
To strike a balance between precision and practicality, consider the following:
- Define clear objectives: Identify the key performance indicators (KPIs) that drive business decisions and prioritize accordingly.
- Quantify the cost of precision: Establish the tradeoff between precision and resources, such as time, money, and personnel.
- Embrace approximation: Recognize that 'good enough' can be, well, satisfactory, especially when speed and agility are paramount.
- Monitor and adjust: Continuously evaluate the effectiveness of analytics initiatives and recalibrate as needed.
- Prioritize transparency: Make sure that analytics outputs are interpretable and actionable, rather than opaque and overly complex.
Measuring ROI on Analytics Investments
Accurate measurement of return on investment (ROI) is vital to justify the financial expenditures on advanced analytics initiatives and to identify areas for optimization. Measuring ROI on analytics investments enables organizations to evaluate the financial impact of their analytics programs and make informed decisions about future investments.
When evaluating the ROI of analytics initiatives, it is essential to take into account both the cost benefit and financial impact. This involves quantifying the costs associated with analytics initiatives, including hardware, software, personnel, and training expenses. It is equally important to assess the benefits, such as increased revenue, improved operational efficiency, and enhanced decision-making capabilities. By calculating the ROI, organizations can determine whether their analytics investments are generating a positive financial impact and identify opportunities for improvement.
A thorough ROI analysis should also consider the intangible benefits of analytics initiatives, such as enhanced customer insights and improved competitiveness. By integrating these factors into the ROI calculation, organizations can gain a more in-depth understanding of the financial impact of their analytics initiatives and make data-driven decisions about future investments.
Frequently Asked Questions
How Do I Ensure Data Quality and Accuracy in My Analytics Efforts?
To guarantee data quality and accuracy, establish a robust data governance framework that outlines clear roles, responsibilities, and protocols for data management. Implement quality metrics to measure and monitor data integrity, completeness, and consistency. Regularly audit and validate data sources, and establish a culture of data accountability. By doing so, you can trust your insights and make informed decisions, ultimately driving business success.
What Is the Ideal Team Structure for an Advanced Analytics Department?
Imagine a conductor leading a symphony orchestra – each musician plays an essential role, but only together do they create harmony. Similarly, an advanced analytics department requires a harmonious team structure. A Centralized Model, where analytics is a standalone department, offers efficiency and consistency. Alternatively, a Decentralized Approach, where analytics is embedded within business units, fosters collaboration and adaptability. The ideal structure depends on the organization's size, complexity, and goals, but ultimately, it's about striking the right chord between specialization and integration.
Can I Use Advanced Analytics With Limited Data or Small Sample Sizes?
When working with limited data or small sample sizes, advanced analytics can still be leveraged to drive insights. Data imputation techniques can fill gaps in data, while statistical relaxation methods can relax assumptions to accommodate smaller samples. By applying these strategies, organizations can still extract value from limited data, ultimately informing business decisions and driving competitive advantage.
How Do I Communicate Complex Analytics Insights to Non-Technical Stakeholders?
Effectively communicating complex analytics insights to non-technical stakeholders is vital for driving business decisions. To achieve this, employ storytelling techniques that convey the narrative behind the data, making it relatable and engaging. Utilize visual aids such as charts, graphs, and heatmaps to simplify complex concepts, ensuring stakeholders grasp key takeaways. By doing so, you'll empower stakeholders to make informed decisions, fostering a collaborative and data-driven environment.
Are There Any Specific Regulations I Need to Consider When Using Advanced Analytics?
When leveraging advanced analytics, it is vital to take into account the regulatory landscape to guarantee compliance. Data privacy regulations, such as GDPR and CCPA, dictate how organizations handle sensitive information. Establishing a robust compliance framework is essential to mitigate risks and avoid penalties. By prioritizing data privacy and adhering to compliance frameworks, organizations can ensure responsible use of advanced analytics and maintain stakeholder trust.